World Models: Infrastructure for the AI-Driven Future
A world model, a digital twin of the world, is a mandatory infrastructure that grounds AI agents in reality, providing the necessary context for reliable autonomous systems.
There's a growing belief within the software community that advanced, generally intelligent AI systems will eliminate the need for traditional software applications. However, even when AI models surpass human intelligence, they cannot reach their full potential without a queryable, structured, real-time, “digital twin” of the world.
Neural networks encode information indirectly through patterns in their weights. They excel at reasoning and generating inferences but aren’t meant to store exact, structured details—such as addresses, financial transactions, or property records within their internal weights.
A world model, a digital twin of the world, is a mandatory infrastructure that grounds AI agents in reality, providing the necessary context for reliable autonomous systems.
World Models
Why Most Industries Lack a Unified World Model
While the creation of a unified, sector-wide data model—a world model—has always been technically feasible, the immense cooperative human effort required to maintain one has outweighed the immediate practical benefits.
Consider the concept of a digital twin, which is widely used in industrial settings like factory floors. A digital twin is a digital representation of physical systems, where real-time sensor data continuously enriches the database. These digital twins enable real-time monitoring, error detection, and more.
However, most sectors aren't factories. In human-centric industries, data often arises from unstructured interactions, with humans themselves acting as the "sensors" responsible for capturing and inputting data into applications.
The ever-evolving world state and the need for normalization, deduplication, and reconciliation make the maintenance of an accurate data repository incredibly labor intensive. Consequently, human-centric sectors have always been fragmented, with specialized applications and CRMs tailored towards each distinct labor type, resulting in data silos. Transferring information across these silos demands additional human effort, leading to communication overhead, duplication, and loss of valuable context.
World Models Enabled by LLMs
The emergence of LLMs enables ingestion of information from any source. As long as the information is stored digitally, it can feed into the world model.
With the advancement in LLMs, it is now possible for a world model to exist where frontline laborers contribute to and benefit from an accurate, comprehensive view of the information landscape. The disparate data silos across organizations, users, and labor types can be unified into a single, cohesive model representing the sector as a whole. Over time, as participation increases and data becomes more comprehensive, this unified model naturally outperforms traditional, fragmented systems.
Real Estate Data
This evolution will occur across many sectors. GoliathData is building it for the world’s largest asset class: real estate.
History of Real Estate Data
The Multiple Listing Service (MLS) serves as the central repository for real estate listings. Whenever a property is marketed to consumers—typically through platforms like Zillow—a licensed agent must first create a listing on the MLS, officially putting it "on the market."
The concept of the MLS dates back to the late 1800s, when real estate brokers gathered at regional association offices to exchange property information. These early networks facilitated deal-making, with brokers compensating one another for helping to close transactions. Over time, formal boards were established, led by members elected from participating brokers. These boards generated revenue through membership dues, subscriptions, and listing fees—creating financial incentives to maintain their independent systems.
Real Estate Data Today
With the rise of the internet, the real estate industry shifted to digital databases. Platforms like Zillow, Realtor, Redfin, and Trulia emerged to aggregate and present a unified view of MLS data. However, the MLS landscape remains fragmented, with over 500 systems operating nationwide—each governed by its own elected board. This decentralization persists largely due to entrenched revenue streams for local boards.
The MLS software used by agents remains severely outdated. For instance, the MLS in NYC uses a platform called MLS Stratus. To list a property, agents must fill out lengthy forms, often completing only the required fields and leaving the rest blank. Many agents outsource this tedious task to overseas VAs. Moreover, MLS Stratus still presents property data as PDFs.
Given the relatively low transaction volume for most agents, they typically rely on memory, texts, emails, and notes rather than comprehensive CRMs.
Estimates suggest that 60–80% of top-performing agents operate solo or in very small teams, minimizing overhead and focusing purely on sales and client relationships. These top agents often rely on referrals, repeat business, and strong brands that generate leads organically, eliminating the need for extensive cold outreach.
This underscores why the existence of a unified real estate world model is not yet a reality. For most top agents, manually logging each transaction detail provides little immediate benefit compared to their informal methods of managing information.
The Future Of Data For Real Estate
The MLS landscape and agents demonstrate one concrete example of why a unified model is not yet a reality. Agents are just one labor type within real estate, alongside title companies, county recorders, appraisers, inspectors, deal sourcing specialists, and brokers who experience the same problems.
With LLMs, we can build a unified world model for the entire real estate sector, enriched through the natural workflows of each laborer (texts, emails, forms, reports). Rather than communicating across fragmented systems, a single shared record representing each property, person, owner, or entity and its complete transaction history is collaboratively maintained and continuously updated. Operators can quickly access accurate, contextual information without tedious coordination across data silos.
Data Layers
We're creating a layered data model structured as a dynamic, interconnected network—a living, breathing data ecosystem—unifying all information directly from primary sources into a single world model.
Probabilistic Layer (Quant Layer): For real estate agents, revenue growth hinges on one critical factor: securing listings or seller leads. Similarly, real estate investors encounter a key profit bottleneck—access to undervalued properties. The sheer number of companies dedicated to providing seller leads demonstrates this need. Ask any agent what they want, and they'll say seller leads. Our approach mirrors quantitative trading—leveraging information asymmetry and predictive insight to identify seller intent and undervalued properties first. By ingesting probabilistic signals derived from scraping and inference, our world model anticipates market movements and act on seller leads early, providing an unmatched competitive advantage
Operational Layer: This captures real-world interactions from agents, brokers, title companies, county recorders, appraisers, inspectors, callers, and others. By breaking down traditional silos, each laborer contributes to and benefits from a unified, comprehensive world model.
Consumer Layer: As we embed into the workflows of frontline laborers and siloed data sources, our world model will organically become richer and more comprehensive. At this stage, we can introduce a consumer-facing layer, one where only licensed operators can input and query data from our system, and AI agents have the necessary data infrastructure to advance.
While foundation models and AI agent architectures continue to evolve and improve, their full potential depends on a robust data infrastructure that accurately mirrors the real world. By building a unified world model—one that integrates probabilistic insights, operational data, and accessible consumer interfaces—we are laying the groundwork for an AI-driven future in real estate where intelligent systems are not only capable of complex reasoning, but also deeply anchored in real-world context.
Written By:

Brian Przezdziecki
Founding Engineer
Ready to connect with homeowners ready to list?
Define your target area, and we'll connect you with home sellers ready to list. No cold calls, no guesswork. Just show up to the appointment, and sign the listing agreement. Pay only when the deal closes.
*You will be subscribe to our newsletter
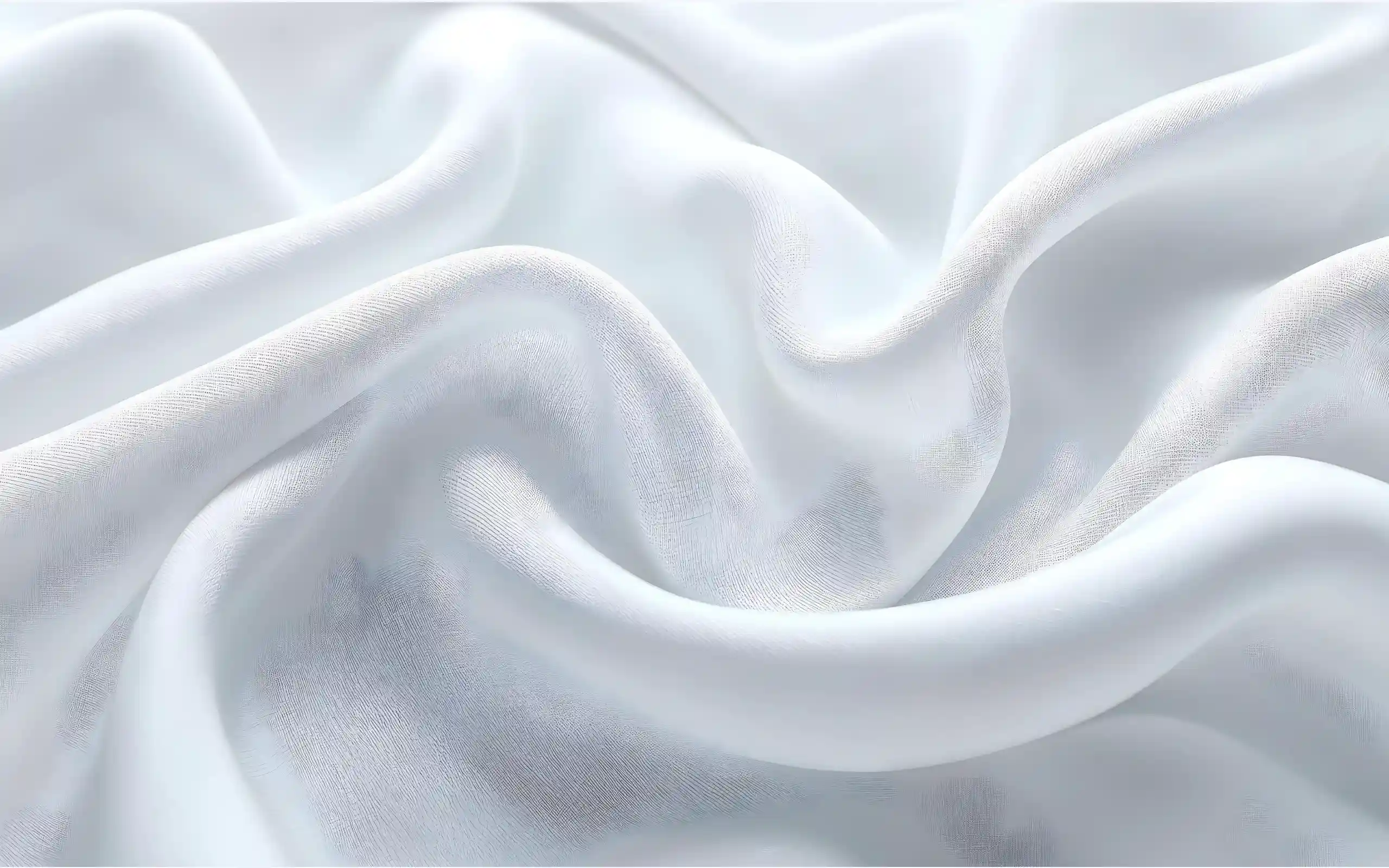